AIOps vs Traditional IT Operations: Comparison for Uptime and Performance
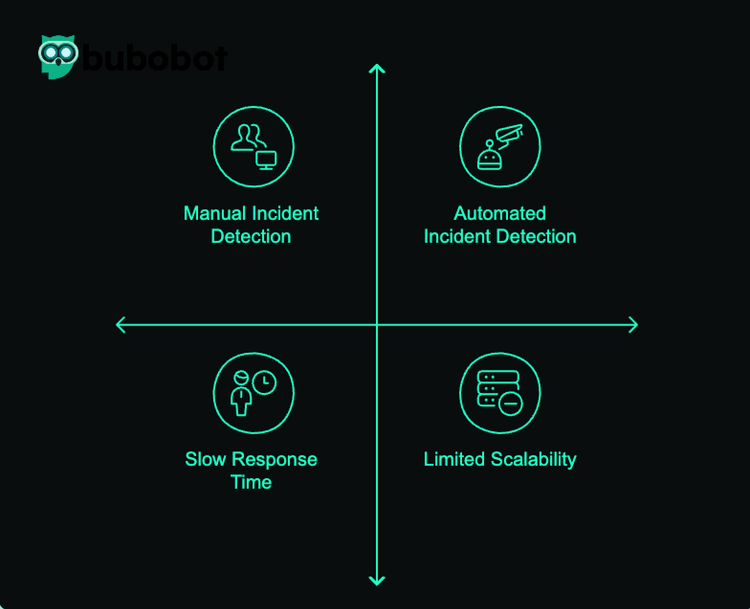
1. Introduction: The Shift from Traditional IT Ops to AIOps
The Growing Complexity of IT Operations
Today's IT environments have outgrown traditional monitoring approaches. As infrastructure expands across clouds, containers, and microservices, manual monitoring and rule-based alerts simply can't keep pace. When systems fail, operations teams often spend hours checking/analyzing through logs from multiple tools to find the root cause—all while the business suffers from costly downtime.
The Need for AI-Driven IT Ops
This growing complexity has conducted to the rise of AIOps (Artificial Intelligence for IT Operations). By applying machine learning and automation to uptime monitoring, organizations can now predict potential failures before they happen, automatically correlate related incidents, and dramatically reduce response times.
As businesses depend increasingly on their digital infrastructure, these AI-powered capabilities aren't just advantageous—they've become essential for maintaining competitive system reliability and performance.
2. AIOps vs. Traditional IT Operations
Understanding the fundamental differences between traditional IT operations and AIOps-driven approaches reveals why organizations are increasingly adopting AI-powered monitoring solutions for critical systems and applications.
Factor | Traditional IT Operations | AIOps |
---|---|---|
Definition | Manual and rule-based approach to monitoring and managing IT infrastructure and services | Integration of AI/ML with IT operations to automate and enhance monitoring, incident response, and decision-making |
Incident Detection | Rule-based alerts triggered by predefined thresholds, leading to reactive responses after issues occur | AI-driven real-time anomaly detection that can identify unusual patterns before they cause service disruptions ✅ |
Response Time | Manual troubleshooting requiring human investigation, resulting in slower resolution | Automated incident response with intelligent remediation suggestions or actions, dramatically reducing MTTR 🚀 |
Scalability | Limited to predefined rules and manual updates as systems grow | Self-learning algorithms that automatically adapt to growing and changing environments 📈 |
Data Processing | Human-driven log analysis with limited ability to process massive datasets | Big Data analytics combined with machine learning for comprehensive insights across vast operational data 🔍 |
Uptime Optimization | Preventive maintenance based on schedules and best practices | Predictive failure prevention through pattern recognition and trend analysis ⚡ |
Resource Utilization | Manual scaling and optimization based on historical usage patterns | AI-optimized automatic resource allocation based on predicted needs and usage patterns 🔄 |
Monitoring Approach | Reacts after failures occur, often detecting issues only after user impact | Predicts potential failures by analyzing subtle system behavior changes before outages happen |
Capacity Planning | Historical data-based forecasting with limited accuracy | AI-powered predictive analytics combining historical trends with business context for precise capacity requirements 📈 |
Application Optimization | Manual performance tuning on scheduled intervals or after incidents | Continuous, automated performance analysis and optimization suggestions based on actual usage patterns ⚙️ |
Key Takeaway: AIOps significantly outperforms traditional IT operations in incident detection speed, response time automation, and proactive issue resolution. While traditional monitoring waits for thresholds to be crossed, uptime monitoring powered by AI can detect anomalies that would otherwise go unnoticed until they cause downtime.
3. Real-World Use Cases & Industry Adoption
AIOps adoption is accelerating across industries as organizations recognize its transformative impact on reliability and performance.
Cloud & SaaS Companies
Cloud service providers and SaaS companies leverage AI-powered monitoring to:
- Implement intelligent auto-scaling that anticipates demand spikes
- Enhance uptime monitoring with predictive failure detection
Companies like Salesforce use AIOps to maintain their 99.9%+ availability commitments while serving millions of users.
More info: https://www.zluri.com/blog/aiops-platforms#5-bigpanda
Financial & E-Commerce Platforms
Financial institutions and e-commerce platforms have embraced AIOps for:
- AI-driven fraud detection integrated with operational monitoring
- Real-time transaction monitoring that ensures payment processing availability
Major payment processors like Mastercard, use AIOps to analyze billions of transactions, automatically detecting performance bottlenecks during peak shopping periods—ensuring website uptime monitoring remains strong even under extreme loads.
More info: https://b2b.mastercard.com/ai-and-security-solutions/brighterion-ai/
Telecom & Enterprise IT
Large telecommunications providers and enterprise IT departments leverage AIOps for:
- Network incident management automation
- Predictive maintenance of physical infrastructure
Vodafone has been public about their AIOps implementation. They deployed an AIOps solution to monitor their network operations, reportedly resulting in a significant reduction in customer-impacting incidents. Their case was documented in several industry publications, where they reported being able to predict and prevent network issues before they affected customers.
More info: https://www.vodafone.com/about-vodafone/what-we-do/artificial-intelligence
4. Conclusion & The Future of AIOps
As IT environments grow increasingly complex, AIOps has become not just an evolution but a necessary revolution in maintaining reliability and performance. The data clearly shows that AI-powered monitoring outperforms traditional methods across all key metrics—from faster incident detection to predictive maintenance and optimized resource utilization.
Looking ahead, AIOps will continue to advance with improvements in machine learning capabilities, greater remediation autonomy, and deeper DevOps integration.