The Rise of AI-Powered Monitoring [UPDATE 2025]
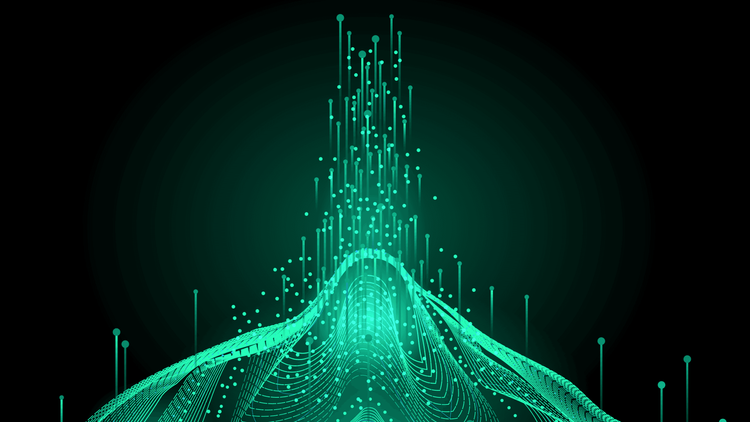
1. Introduction
As IT systems grow more complex, traditional tools struggle to keep up. Distributed architectures, microservices, and multi-cloud environments demand smarter uptime monitoring solutions to prevent downtime, protect revenue, and reduce operational costs. Reactive tools leave DevOps teams overwhelmed, consuming valuable time and manpower on avoidable incidents.
AI-powered monitoring offers proactive, adaptive solutions to minimize effort, enhance reliability, and safeguard user trust. By predicting issues and automating responses, AI monitoring tools save time, reduce effort, and minimize the need of manual intervention—all while safeguarding business performance.
With Bubobot’s AI-powered monitoring, businesses can move from detection to prevention, reducing the financial impact of incidents and freeing teams to focus on strategic growth.
2. The Limitations of Traditional Monitoring
Traditional monitoring tools struggle in today’s complex IT environments. Reactive monitoring, static thresholds, and alert fatigue overwhelm teams, delay incident resolution, and increase the risk of downtime. These limitations hinder operational efficiency, waste resources, and leave systems vulnerable to preventable issues.
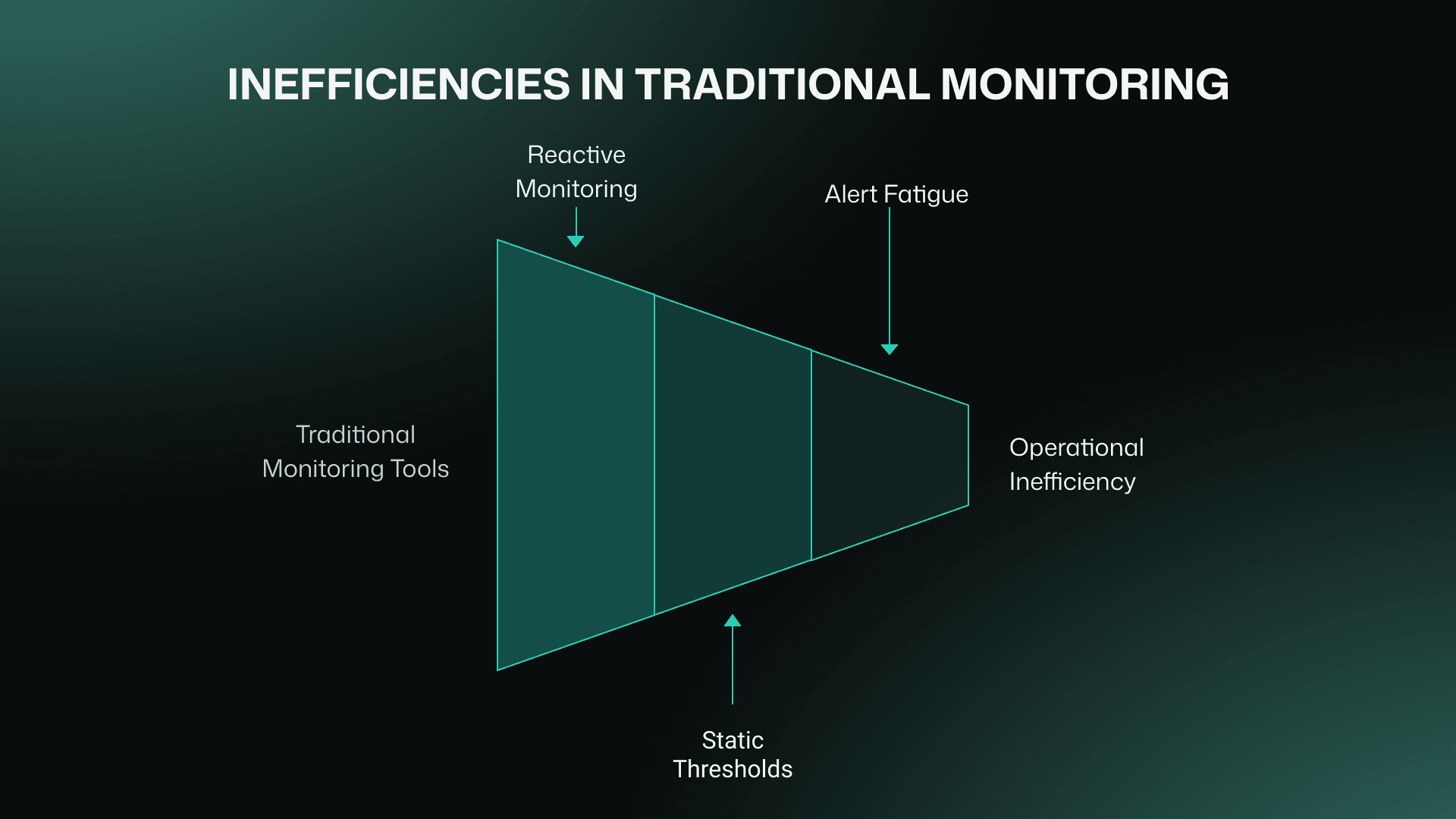
Here are the key limitations of traditional monitoring systems:
Reactive Monitoring
Reactive monitoring detects and reports issues only after they occur, forcing IT teams to focus on firefighting rather than prevention.
Why It’s Critical
- Missed Opportunities: Without proactive insights, teams cannot address early warning signs that could prevent incidents.
- Extended Downtime: By the time an issue is detected, it has often already impacted systems, leading to costly outages.
- Resource Drain: Teams are forced into a reactive mode, expending valuable time and effort on incident recovery rather than innovation.
Static Thresholds
Static thresholds in traditional tools can’t adapt to dynamic workloads, causing false positives or missed critical alerts.
Why It’s Critical
- False Positives: Predictable events like marketing campaigns or seasonal spikes trigger irrelevant alerts, creating unnecessary panic.
- Missed Critical Alerts: Unusual patterns, such as slow response time during peak hours, may not trigger an alert if they fall within predefined thresholds.
- Team Fatigue: Static systems create unnecessary workload for teams who must manually adjust thresholds to match changing conditions.
Alert Fatigue
Traditional monitoring tools flood teams with irrelevant alerts, making it hard to identify critical issues among the noise.
Why It’s Critical
- Missed Critical Alerts: High-priority incidents can get buried under irrelevant notifications, delaying response times.
- Team Burnout: Alert fatigue demoralizes IT teams, reducing their efficiency and increasing the risk of errors.
- Slower Resolutions: Teams waste valuable time triaging unnecessary alerts instead of addressing the root cause of incidents.
Example
The graph below illustrates the challenges of traditional monitoring tools during a flash sale, where response time spikes dramatically. Traditional systems often misclassify expected traffic surges as anomalies, generating irrelevant alerts that overwhelm teams. Meanwhile, critical performance bottlenecks are overlooked in the noise, delaying resolution and increasing the risk of downtime.
Response time spikes during a flash sale highlight the limitations of static thresholds in traditional monitoring tools. AI-powered tools adapt dynamically, filtering noise and prioritizing critical anomalies.
3. What Can AI-Powered Monitoring Solve?
As traditional monitoring tools fail in today’s dynamic environments, AI-powered monitoring emerges as the solution to these challenges. By leveraging advanced analytics and machine learning, AI monitoring tools provide DevOps teams with a flexible alert system that focuses on what is truly necessary.
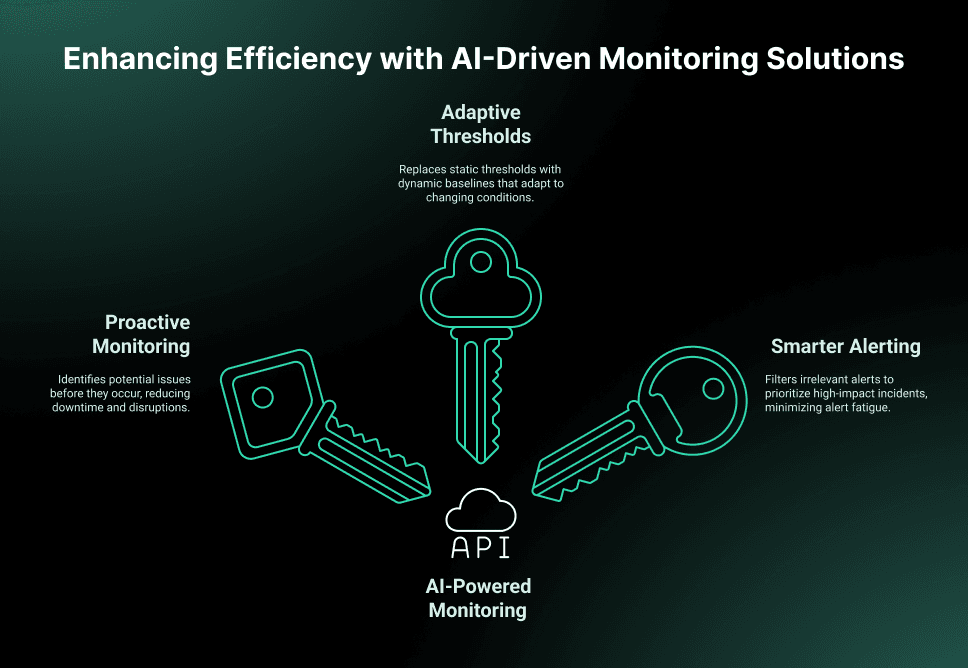
Here’s how AI-powered monitoring tackles the challenges of traditional systems:
Proactive Monitoring
What is it?
AI-powered monitoring moves beyond reactive detection by identifying patterns and anomalies that signal potential issues before they occur.
How does it help?
Teams can address problems proactively, reducing downtime, preventing disruptions, and shifting focus from firefighting to innovation.
Adaptive Thresholds
What is it?
AI replaces static thresholds with dynamic, data-driven baselines that adapt to changing workloads and conditions.
How does it help?
Reduces false positives during predictable events and ensures critical anomalies are detected even in complex, dynamic environments.
Smarter Alerting
What is it?
AI-powered monitoring uses contextual analysis to filter irrelevant alerts and prioritize high-impact incidents.
How does it help?
Minimizes alert fatigue, ensures teams focus on pressing issues, and speeds up incident resolution by highlighting root causes.
4. Conclusion
AI-powered web uptime monitor solutions have revolutionized how DevOps and IT teams manage complex systems. By addressing the core limitations of traditional monitoring tools, AI monitoring tools empower organizations to achieve:
- Stay Ahead with Proactive Monitoring: AI-powered monitoring tools help detect and resolve issues before they escalate, keeping operations smooth and minimizing costly downtime.
- Adapt to Change with Dynamic Thresholds: Say goodbye to static rules – AI adjusts to your workload in real-time, reducing unnecessary alerts and catching critical issues when they matter most.
- Cut Through the Noise with Smarter Alerts: AI filters out irrelevant notifications, ensuring your team only receives alerts that require immediate attention. This boosts efficiency and prevents burnout.
By leveraging advanced AI-powered monitoring tools, organizations can optimize resources, enhance system reliability, and maintain user trust. Bubobot’s solutions are designed to help IT teams work smarter with proactive monitoring, adaptive thresholds, and a flexible alert system tailored to today’s IT complexities.
5. How Bubobot can for uptime monitoring?
As a leading web uptime monitor, Bubobot is not just another monitoring tool—it's an AI-powered solution designed to revolutionize incident management. At the heart of Bubobot is its advanced anomaly detection engine, a feature that sets it apart from traditional solutions.
Bubobot’s anomaly detection continuously analyzes historical and real-time data, enabling proactive responses to potential issues, adaptive thresholds, and smarter alerts. Here’s how it solves critical monitoring challenges:
Proactive Monitoring
What Bubobot Offers: Proactive anomaly detection built into its AI engine. Bubobot continuously analyzes historical and real-time data to identify patterns, detect anomalies early, and flag potential issues before they escalate.
How It Helps: This ensures that teams can address problems preemptively, reducing downtime and maintaining smooth operations.
Adaptive Thresholds for Dynamic Workloads
What Bubobot Offers: AI-based adaptive thresholds that evolve with your system’s workload. Bubobot automatically adjusts to dynamic conditions, eliminating static thresholds that lead to false positives and missed alerts.
How It Helps: Bubobot allows routine events, like flash sales, to pass as expected behavior while flagging unusual anomalies that demand attention.
Smarter Alerts to Solve Alert Fatigue
What Bubobot Offers: Contextually intelligent alerts powered by AI. Bubobot reduces noise by filtering out irrelevant notifications and prioritizing critical incidents that require immediate action.
How It Helps: Teams stay focused on key issues, avoid burnout, and resolve incidents faster with less effort.
Ready to simplify your incident management? Try Bubobot’s AI-powered monitoring tools and experience the difference between smart alerts and anomaly detection.