Why AI is Key for Incident Prevention
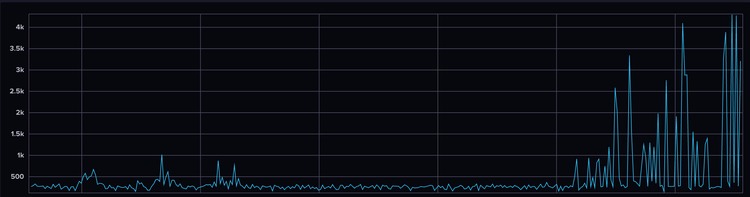
1. Introduction
According to recent research, the average cost of downtime has inched as high as $9,000 per minute for large organizations. For higher-risk enterprises like finance and healthcare, downtime can eclipse $5 million an hour in certain scenarios—and that’s not including any potential fines or penalties — Forbes Could AI be the key to staying ahead of downtime?
Incidents caused by system failures, resource spikes, or unforeseen events can disrupt operations, damage user trust, and incur significant costs. Proactively preventing these incidents is crucial for maintaining business continuity, minimizing revenue loss, and protecting company reputation.
Why Preventing Incidents Matters
Preventing incidents is about more than just reducing downtime—it’s about enabling long-term success:
- Revenue Protection: Downtime directly impacts sales, transactions, and service delivery, hitting a company’s bottom line.
- User Trust and Retention: Frequent disruptions erode customer confidence, driving them to competitors and damaging brand reputation.
- Operational Efficiency: Reactive firefighting drains IT resources, wastes time, and diverts focus from strategic initiatives.
- Regulatory Compliance: Industries like healthcare and finance face strict uptime requirements. Failures can lead to costly penalties.
However, traditional incident management tools often fall short due to their static thresholds, alert noise. AI for incident prevention revolutionize detection and response, ensuring smarter, proactive solutions.
In this article, we’ll explore why incident prevention is critical for business success, explore the limitations of traditional monitoring tools, and uncover how AI-driven solutions are transforming incident management.
2. Critical Impacts of Incident
On Business
Incidents cause unplanned downtime, halting operations and making critical services unavailable. For businesses, this directly translates into financial losses, missed opportunities, and reduced customer satisfaction.
On User Trust
Incidents ruin user confidence and trust in your service. Frequent outages or degraded performance push users to seek alternatives, impacting customer loyalty. Additionally, negative experiences spread quickly, damage your brand’s reputation and making it harder to retain or attract new customers.
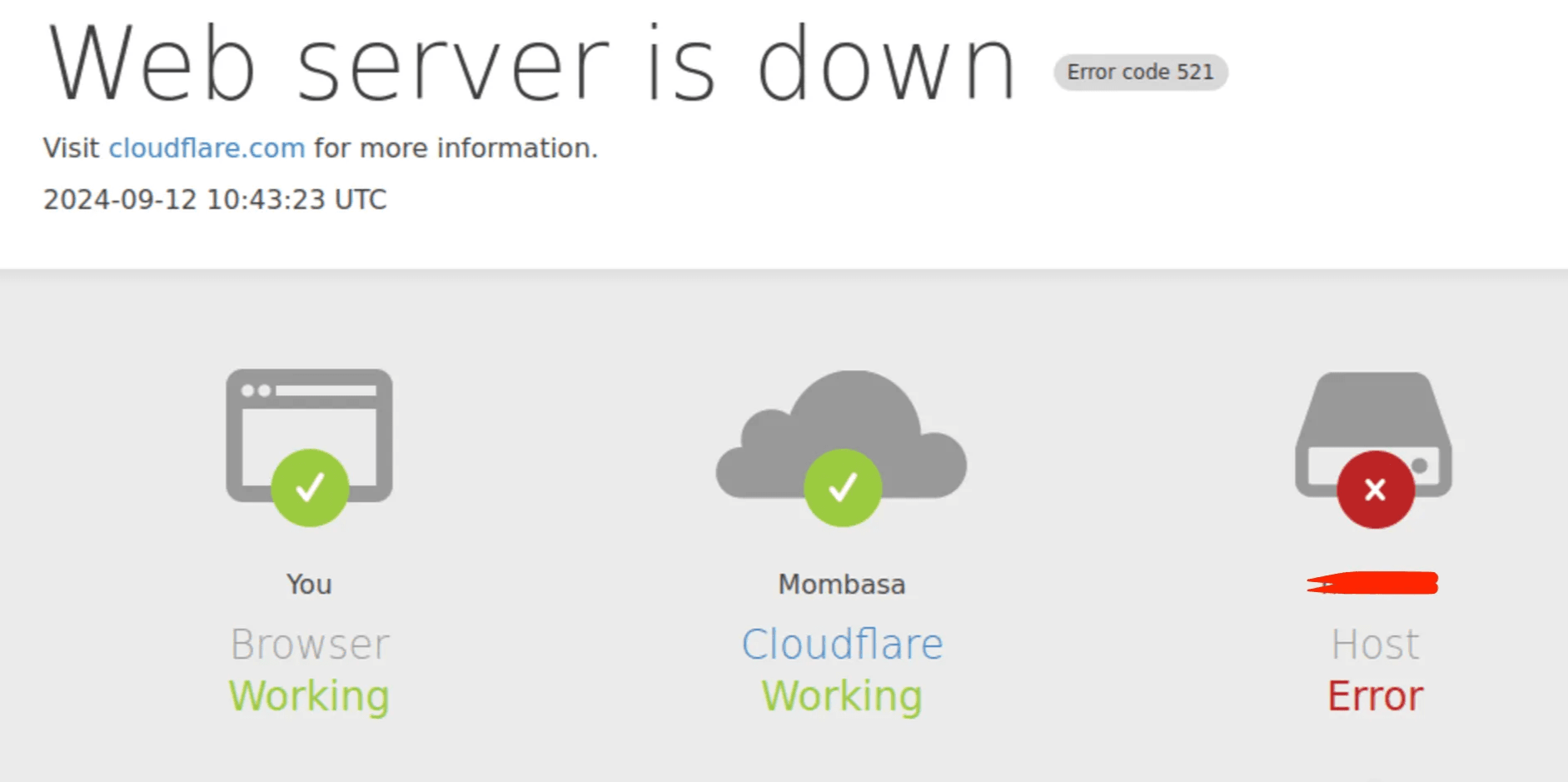
On DevOps and IT Teams
Frequent incidents can lead to prolonged recovery times, especially when entire systems are impacted. This not only delays strategic projects but also fuels frustration, burnout, and inefficiencies in resolving critical issues, leaving teams feeling overwhelmed and unproductive.
These critical impacts—financial losses, eroded user trust, and strained IT resources—highlight the urgency of preventing incidents before they occur. However, traditional incident management tools often fail to provide the proactive capabilities needed to address these challenges effectively.
3. The Disadvantages of Traditional Monitoring Solutions
Despite their widespread use, traditional monitoring tools struggle to maintain effective downtime monitoring, and keep up with the complexities of modern IT systems. Here’s why:
Lack of Foresight
Traditional systems detect issues only after they occur, providing no opportunity for proactive intervention. This reactive approach delays resolution and increases downtime, leaving teams scrambling to fix problems after the damage has been done.
Example: A server’s memory usage steadily increases overnight, causing an unplanned crash. Without predictive monitoring, the team is blindsided, resulting in extended downtime.
Alert Fatigue
Traditional monitoring tools often generate a flood of alerts, overwhelming teams with irrelevant notifications. This constant barrage of information makes it difficult to focus on critical issues, causing high-priority alerts to be missed amidst the noise.
Example: During a server outage, hundreds of low-priority alerts obscure the root cause, delaying resolution and extending downtime, and compromising downtime monitoring efforts.
Static Thresholds
Traditional tools rely on pre-set, static thresholds that fail to adapt to dynamic workloads. This results in false positives during predictable traffic spikes or missed warnings in unexpected scenarios. Example: During the launch of a new cryptocurrency, an exchange experiences an unprecedented surge in traffic as users rush to trade. Outdated thresholds flag the surge as an anomaly, triggering irrelevant alerts and overwhelming the operations team, even though the traffic increase is expected.
These challenges—delayed detection, overwhelming alerts, and inflexible thresholds—highlight the need for intelligent, proactive solutions to effectively prevent incidents.
4. Why AI is Essential for Incident Prevention?
AI-powered monitoring revolutionizes incident prevention by addressing these shortcomings, empowering teams to stay ahead of potential issues while improving efficiency and system reliability.
Proactive Monitoring
- What It Solves: Reactive detection
- How It Works: AI analyzes historical and real-time data to identify trends and anomalies, predicting incidents before they occur, enhancing uptime monitoring capabilities. This proactive approach minimizes downtime and ensures smoother operations.
- Example: AI identifies a sudden and dramatic increase in response time for an API endpoint. Based on historical trends, it predicts that this could lead to unreachability or timeout errors if not addressed. The team takes preemptive action by optimizing the backend service, preventing downtime and maintaining user experience.
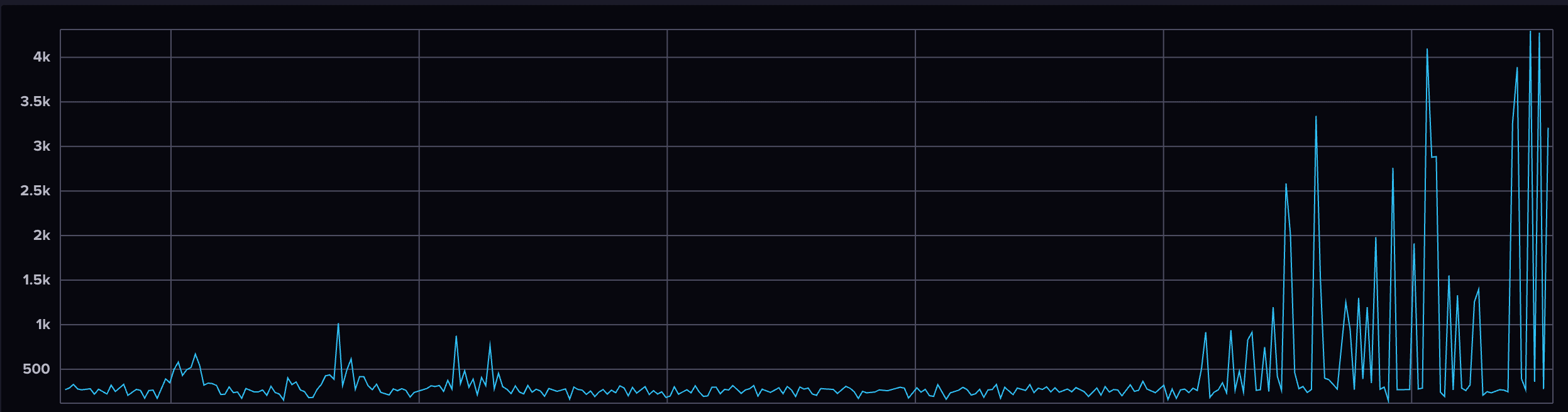
AI-powered monitoring detects this anomaly by comparing it to historical response time patterns, enabling teams to take proactive action before downtime occurs
Smarter Alerts
- What It Solves: Alert overload
- How It Works: AI filters irrelevant alerts and prioritizes critical incidents based on contextual analysis, ensuring teams focus on high-impact issues while reducing noise.
- Example: During a traffic spike, AI suppresses low-priority alerts while highlighting an unusual spike in error rates, enabling quicker root cause analysis.
AI-Based Thresholds
- What It Solves: Static thresholds
- How It Works: AI replaces fixed thresholds with adaptive ones that evolve based on workload patterns and real-time conditions, eliminating false positives and missed warnings.
- Example: During a flash sale, AI recognizes high traffic as normal but flags an abnormal surge in failed transactions, prompting immediate action.
AI-powered monitoring transforms incident prevention by shifting from reactive to proactive practices, reducing alert fatigue, and adapting dynamically to changing conditions
5. Explore More About Incident Management with AI
If you’re looking into how AI-powered monitoring tools are revolutionizing incident management, check out our previous blog post, The Rise of AI-Powered Monitoring. This comprehensive guide explores the evolution of monitoring tools and how AI for incident prevention addresses the challenges faced by modern DevOps and IT teams.
6. Conclusion
Why is AI the key to incident prevention? Traditional monitoring tools fall short in today’s dynamic IT environments, leaving organizations reactive and vulnerable to costly downtime. By integrating AI-powered monitoring tools, businesses can take a proactive approach to incident management, ensuring operational resilience and user satisfaction.
AI empowers teams with proactive monitoring to identify and address anomalies before they escalate, a flexible alert system to minimize noise while ensuring critical alerts are always prioritized, and dynamic insights that enhance operational efficiency. These features make AI incident tools an essential tool for modern organizations to prevent incidents effectively.
Bubobot’s AI-driven tools take this a step further by offering:
- Anomaly Detection: Identify unusual patterns across metrics to prevent incidents before they escalate.
- Dynamic Thresholds: Automatically adjust thresholds to evolving workloads, minimizing false positives.
Additional features to enhance Incident Management:
- Escalation Policies: Ensure critical issues are addressed promptly by the right team members.
- Multi-Channel Alerts: Bubobot ensures that critical alerts are delivered to the right teams in real-time through a wide range of integrations.
Ready to experience smarter incident prevention? Try Bubobot’s AI incident tools today and take control of your system’s reliability with advanced anomaly detection and proactive insights!